Introduction to Generative AI
Generative AI refers to a subset of artificial intelligence technologies that have the ability to create content. This can include images, text, music, and even computer code. These systems learn from vast datasets to generate new content that is often indistinguishable from content created by humans.
At the heart of Gen AI is machine learning, particularly deep learning networks, which analyze and reproduce complex patterns and sequences. The applications of generative AI are growing rapidly, touching industries such as entertainment, healthcare, and automotive, where they are used to innovate and streamline processes.
As Gen AI continues to evolve, it plays a crucial role in driving technological advancement and digital creativity.
How does Generative AI work?
Basics of Generative AI
Gen AI refers to a subset of artificial intelligence technologies that can generate new content, whether it be images, text, or other media forms, by learning from existing data. Unlike traditional AI models that are primarily used to analyze and interpret data, generative AI goes a step further by creating brand new data instances that maintain plausible attributes of original datasets.
This capability is frequently built upon machine learning frameworks, particularly utilizing deep learning neural networks. At its core, generative AI operates by identifying and learning the patterns and distributions in a dataset and then using these findings to generate similar but distinct outputs.
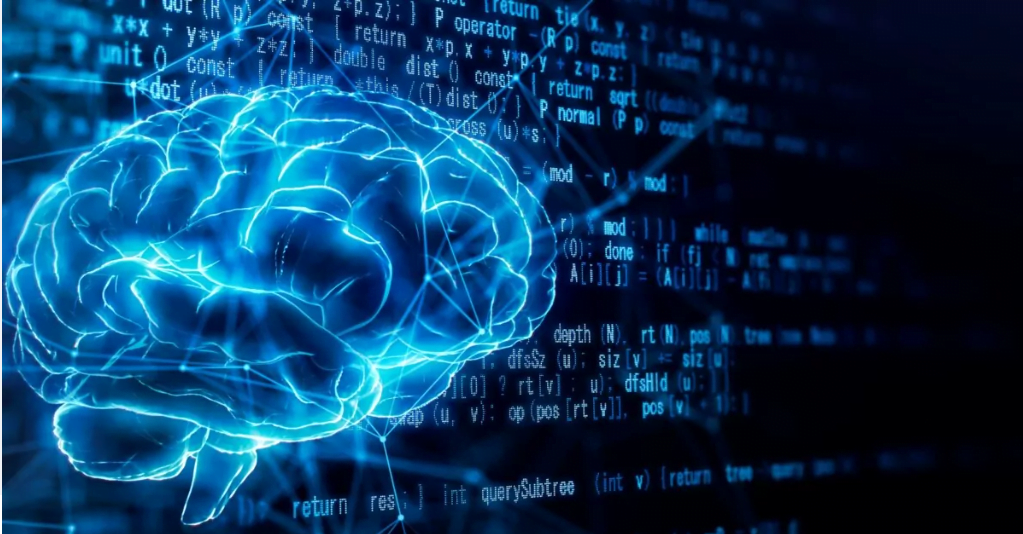
Components of Generative AI algorithms
Gen AI algorithms predominantly rely on two main architectures: Generative Adversarial Networks (GANs) and Variational Autoencoders (VAEs).
– Generative Adversarial Networks (GANs) involve two neural networks, the generator and the discriminator, which work in opposition to each other. The generator produces samples aimed at mimicking the training data, while the discriminator evaluates them against the true data, learning to differentiate between the two. This competition drives the generator to improve its productions until the discriminator can no longer separate the generated data from the actual data.
– Variational Autoencoders (VAEs), on the other hand, encode input data into a condensed representation and then reconstruct it back to its original form. During this process, the model learns the parameters of the probability distribution that represents the data. VAEs are particularly useful in modeling complex data distributions and generating new data points with varied attributes.
Applications of Generative AI
In art and design
In the realms of art and design, generative AI has been a game changer, enabling artists and designers to push the boundaries of creativity. Artists use tools powered by generative AI to create complex and intricate designs that would be extremely time-consuming or even impossible to produce manually
. For instance, AI-driven algorithms can analyze historical art styles to generate paintings that blend multiple genres or create entirely new aesthetic styles. Furthermore, in graphic design, AI tools can automatically generate layouts, color schemes, and typographies, streamlining the creative process and allowing designers to focus on more strategic elements.
In healthcare
Generative AI has significant applications in the healthcare sector, specifically in drug discovery and personalized medicine. By modeling biological and chemical interactions, AI can generate predictions for how new drugs might perform, speeding up the R&D process.
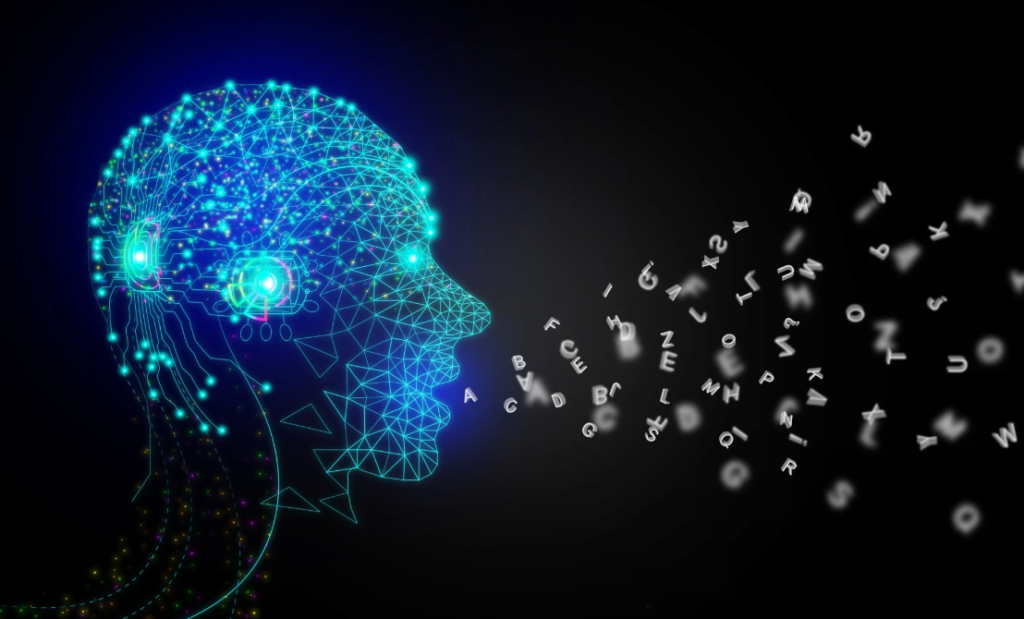
Moreover, generative models can help in synthesizing medical data for research, ensuring patient privacy is maintained by creating anonymized datasets for studies. Personalized medicine also benefits from generative AI by tailoring treatments based on the predicted outcomes generated by AI analysis of patient-specific data.
In media and entertainment
The media and entertainment industry has embraced generative AI to produce content at scale. In journalism, AI tools can generate drafts or entire articles based on data inputs, allowing for rapid response times in news reporting.
In film production, AI can be used to create realistic CGI characters and environments, reducing costs and production time. Moreover, the music industry uses AI to compose music or enhance sound quality, providing artists with new tools to express their creativity.
Gen AI not only assists in content creation but also helps in personalizing user experiences by recommending content based on individual preferences learned over time. These applications of generative AI enhance not only efficiencies but also the reach and accessibility of creative content.
Impact of Generative AI
Advantages-
Gen AI has rapidly become a transformative technology across various sectors, offering numerous advantages that drive innovation and efficiency. Key among these benefits is its ability to create new content, from realistic images and videos to unique textual materials and complex data sets.
This capability is particularly valuable in fields such as digital marketing, where personalized content is crucial for customer engagement. Additionally, Generative AI excels in automating the design process in industries such as fashion and manufacturing, significantly reducing the time and cost involved in product development.
Another major advantage is its application in data augmentation, helping to improve machine learning models’ accuracy without the need for extensive manual labor to gather new data.
Challenges and limitations-
Despite its numerous benefits, Gen AI also faces significant challenges and limitations that need addressing. One of the primary concerns is the quality of output, which can be unpredictable and vary greatly depending on the training data and algorithms used.
There is also the issue of computational costs, as training generative models often requires substantial resources, making it less accessible for smaller businesses and organizations. Moreover, the potential for misuse, such as creating deepfakes, raises serious ethical and security concerns that must be carefully managed.
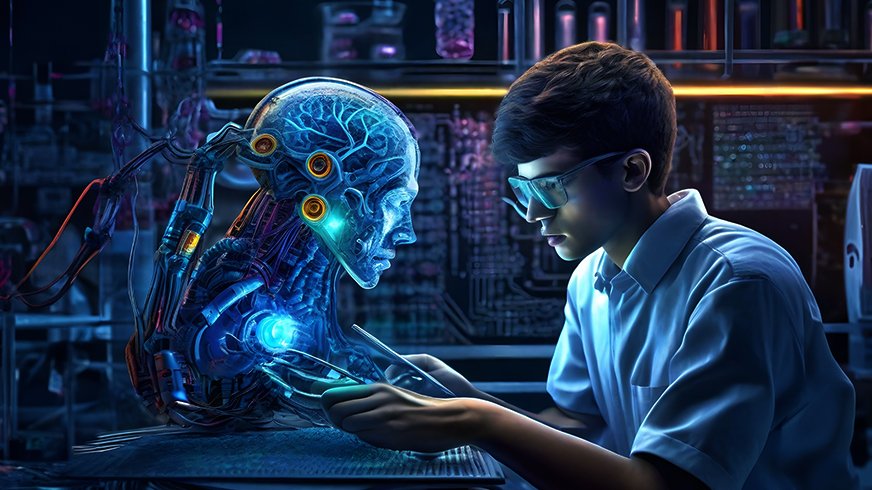
Another limitation is the current lack of understanding in some areas of how these AI models make specific decisions, which can lead to trust and reliability issues among users.
Future prospects of Generative AI technology
Looking forward, the prospects of Generative AI technology are vast and promising. Continuous advancements in machine learning and hardware are expected to address many of the current limitations, such as reducing the costs and improving the quality and stability of AI-generated outputs.
Additionally, as researchers and developers gain a better understanding of the technology’s mechanisms, we’re likely to see more reliable and trusted applications emerging. Generative AI is also set to revolutionize more industries, including healthcare, where it can be used to simulate medical scenarios for training purposes or to generate personalized treatment plans.
The technology’s ability to enhance human creativity and to create safe, virtual testing environments holds significant potential for future growth and innovation.
Ethical Considerations in Generative AI
Bias and fairness issues
One of the primary ethical concerns in Generative AI revolves around bias and fairness. Since AI systems learn from data provided to them, any inherent bias in the data will likely be reflected in the outputs generated by AI. This can perpetuate or even exacerbate existing prejudices in sensitive areas such as recruitment, law enforcement, and loan approval processes.
Addressing these issues requires rigorous auditing of data sets for biases, development of more robust algorithms that can identify and correct biases automatically, and continuous monitoring of AI behavior to ensure fairness and equity.
Privacy concerns
Generative AI also poses significant privacy concerns, particularly regarding how personal data is used to train these systems. The technology’s capability to analyze and reproduce human-like outputs can lead to unauthorized uses of individuals’ data, potentially leading to identity theft or misuse of personal information.
To mitigate these risks, it is important to establish strict data handling and confidentiality protocols, obtain clear consent from data subjects, and apply state-of-the-art data anonymization techniques before using personal data in training AI models.
Regulations and guidelines for Generative AI
The fast pace of development in gen ai has led to calls for more comprehensive regulations and guidelines to ensure the responsible deployment of this technology. Governments and international bodies are beginning to draft policies aimed at protecting individuals and societies from the potential risks associated with AI while promoting its beneficial uses.
Key aspects of these regulatory efforts include ensuring transparency and accountability in AI systems, setting standards for ethical AI deployment, and fostering a collaborative environment where policymakers, technologists, and the public work together to guide the development of gen ai technologies in a way that maximizes societal benefits while minimizing potential harms.
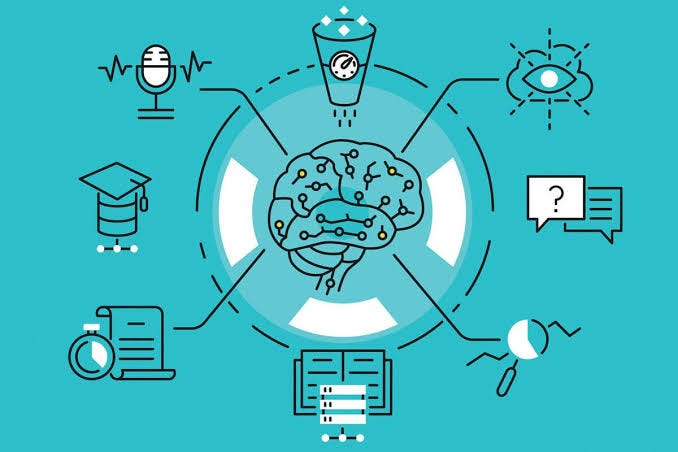
Latest Trends in Generative AI
Innovations in Generative AI technology
Recent breakthroughs in Generative AI technology have been transformative, pushing the boundaries of what machines can create and solve. Improved algorithms and increased computational power have enabled more sophisticated neural networks, which can learn to produce content such as images, audio, and text with astonishing accuracy.
This advancement includes deep learning models like GPT (Generative Pre-trained Transformer) and DALL-E, which can generate human-like text and create images from textual descriptions respectively. Another innovation is the enhancement in reinforcement learning, where AI learns optimal actions through trial and error to maximize the notion of cumulative reward. This has significant implications for automating decision-making processes in complex environments.
Industry applications of the latest Generative AI advancements
The latest advancements in Gen AI are being utilized across various sectors, each reaping the benefits of these new capabilities in unique ways:
– Healthcare: AI algorithms are used to generate synthetic data for training models, ensuring patient privacy while providing ample data for research. Additionally, generative models help in drug discovery by predicting molecular reactions and effectiveness.
– Automotive: In the automotive industry, generative models simulate road scenarios for autonomous vehicle training, significantly reducing the need for real-world testing and enhancing safety features.
– Entertainment: AI-driven tools are generating scripts, composing music, and even creating digital art, transforming how content is created and consumed.
– Manufacturing: Generative design powered by AI allows engineers to create thousands of design alternatives to optimize for materials, functionality, and cost efficiency.
These developments mark just the beginning of widespread transformative impacts that Generative AI is poised to have across the globe, continually redefining the possibilities and applications of artificial intelligence.
Conclusion and Future of Generative AI
Gen AI continues to redefine the boundaries of what machines can achieve, impacting numerous sectors from healthcare to entertainment. As we continue to refine these systems, their integration into daily applications appears more promising and pervasive. The technology’s advancement is driven by the ongoing improvement in algorithms, increase in computational power, and enhanced data collection methods.
Looking to the future, we can anticipate even more sophisticated applications emerging. Generative AI is expected to enable:
– Enhanced customization and personalization in products and services.
– Improved efficiency in industries like manufacturing and logistics through predictive and adaptive AI.
– Revolutionary changes in creative fields such myriads of AI-generated content with implications for artists and legal systems.
However, these advancements accompany ethical considerations and regulatory challenges that need careful management. The balance between leveraging generative AI’s benefits and protecting individual privacy and rights will be crucial. With thoughtful oversight, the future of generative AI promises remarkable transformations across all facets of society.