The Retrieval Augmented Generation (RAG) method offers a potential enhancement in how Generation AI retrieves information, offering businesses a more dependable solution for client interactions.
With the rise of generative AI (GenAI) systems, businesses are eager to incorporate them into various aspects of their operations for optimization. These systems can be utilized in customer service chatbots, intelligent assistants, and domain-specific research tools, among others.
However, the issue of accuracy has been observed in some GenAI models. This calls for the improvement of these systems’ accuracy, relevance, and reliability, enabling businesses to confidently implement them in critical real-world applications.
To address this challenge, a promising solution has emerged known as Retrieval Augmented Generation (RAG). RAG is an innovative technique that enhances the capabilities of language models by integrating them with context-specific data. This integration significantly enhances the accuracy, relevance, and reliability of AI-generated responses.
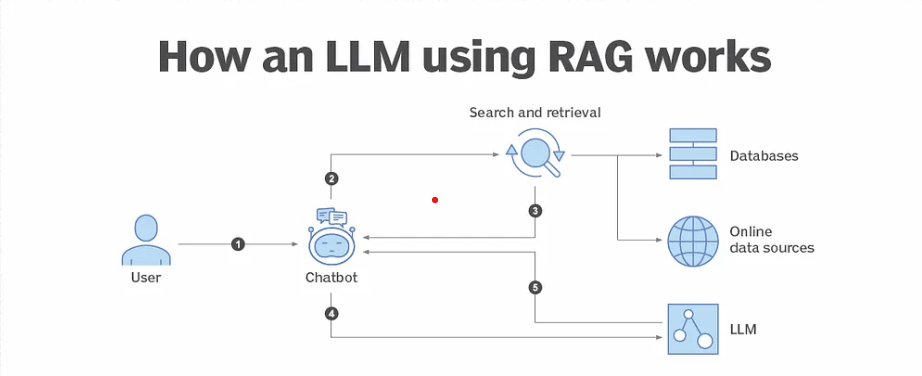
Siddharth Rajagopal, Chief Architect, EMEA at Informatica, explains that RAG combines static LLMs with context-specific data to act as a knowledgeable aide. It matches query context with specific data from a comprehensive knowledge base. For instance, if a customer wants to know about a specific fashion item’s latest trends and pricing, Retrieval Augmented Generation (RAG) can access recent fashion magazines, retail websites, or online reviews to enhance the LLM and provide up-to-date information on product trends, availability, and pricing.
Shane McAllister, Lead Developer Advocate (Global) at MongoDB, simplifies the concept by stating that Retrieval Augmented Generation (RAG) is a process that enhances the accuracy, currency, and context of large Language Models (LLMs) like GPT-4. LLMs are already trained on vast amounts of public data, but RAG can expand these capabilities to private data and specific domains, such as industries or organizations.
RAG systems improve accuracy and currency by incorporating relevant data from external sources, tailoring LLM responses to specific needs with domain-specific data.
Retrieval Augmented Generation (RAG) is ideal for applications that demand precise and informed answers. Adam Lieberman, Chief AI Officer at Finastra, highlights that RAG is well-suited for search and discovery query-based applications where precise and informed answers are essential. This includes customer support in financial applications, medical or legal research, as well as education and tutoring.
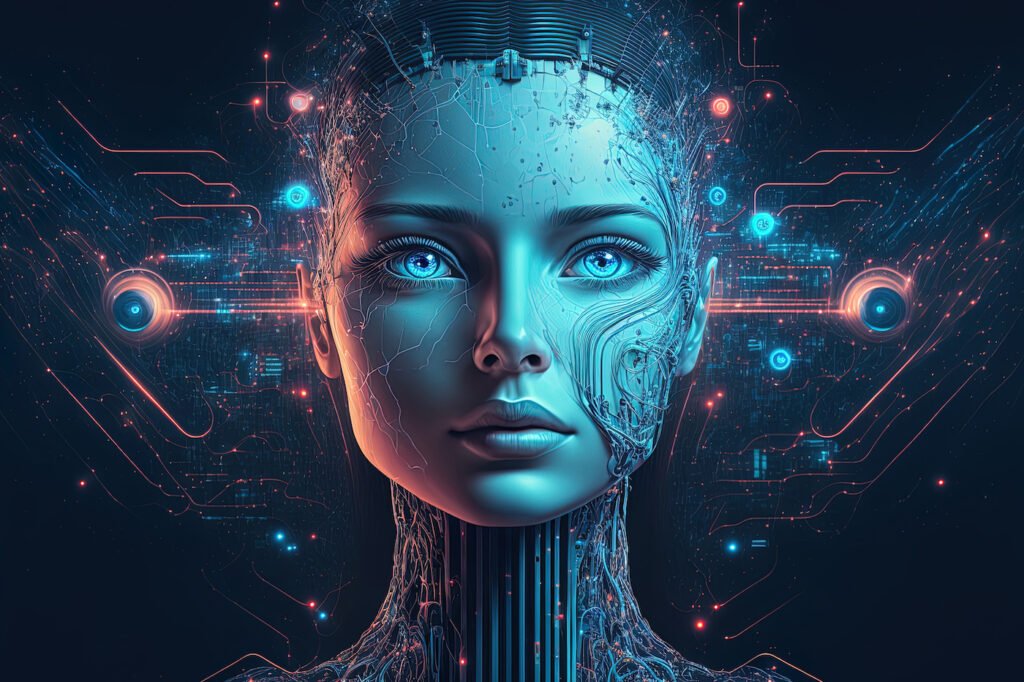
Nevertheless, the capabilities of Retrieval Augmented Generation (RAG) rely on the strength of other technologies.
Maxime Vermeir, Senior Director of AI Strategy at ABBYY, emphasizes the importance of RAG in addressing the challenges of AI hallucinations. He highlights that RAG, enabled by technologies like NLP and Purpose-Built AI, provides access to a highly structured and consistent knowledge base. Vermeir believes that RAG plays a crucial role in enhancing the reliability and contextual awareness of GenAI.
To tackle the issue of AI hallucinations, RAG offers effective solutions. McAllister acknowledges the negative impacts of LLMs hallucinating and generating incorrect information. However, Retrieval Augmented Generation (RAG)’s ability to deliver accurate and up-to-date information helps overcome these challenges.
Joe Mullen, Director of Data Science & Professional Services at SciBite, explains that LLMs tend to hallucinate due to their reliance on outdated information. This can lead to security and privacy vulnerabilities. Mullen suggests that grounding AI can combat these issues.
David Colwell, VP of AI and ML at Tricentis, highlights that RAG addresses the problem of hallucinations by combining the power of LLMs with external knowledge sources. By utilizing various text-based documents and data sources, RAG generates more accurate and informative responses.
By leveraging current and relevant data, RAG reduces the likelihood of hallucinations and enhances the reliability of AI responses.
However, Retrieval Augmented Generation (RAG) does face challenges. One major challenge lies in the misconception surrounding its nature, which can hinder its implementation and wider adoption. Adam Lieberman, Chief AI Officer at Finastra, clarifies three common misconceptions about RAG. Firstly, RAG is not solely a generative model but a technique that combines retrieval and generation. Secondly, while RAG improves response accuracy, there can still be errors in retrieval that result in incorrect or misleading outputs.
Maxime emphasizes that ensuring real-time data retrieval without any delay and maintaining a highly accurate knowledge base are major obstacles. This is a critical aspect because the entire foundation of Retrieval Augmented Generation (RAG) relies on providing relevant and up-to-date information to enhance the output of the language model. If there is a significant delay in retrieving data from the knowledge base, it defeats the purpose of having a real-time retrieval component.
However, advancements in technologies such as Forward-Looking Active Retrieval Augmented Generation (FLARE) and Retrieval-Augmented Customization (REACT) are expected to address these challenges and further improve the capabilities of RAG.
As the adoption of GenAI in enterprises continues to increase, RAG represents a significant advancement in enhancing the accuracy, relevance, and reliability of AI-generated responses.
By integrating Language and Learning Models (LLMs) with context-specific data, RAG systems can provide more informed and up-to-date answers, making them invaluable tools in various applications and industries.
While there are still challenges to overcome, the potential benefits of Retrieval Augmented Generation (RAG), which will grow alongside advancements in new technologies, in improving GenAI systems are significant. Therefore, this technology is poised to play a crucial role in the capabilities of Gen AI systems.